The promise of artificial intelligence (AI) is simple: greater product development efficiency and faster time to market. Cars are becoming more complex and yet engineering teams don’t have more time. By using test data to train self-learning models, today’s visionary engineering leaders are reducing testing time and simulations for products with even the most intractable physics, like electric vehicle (EV) batteries, increasing competitiveness and speeding up time to market.
“Battery independence at any cost”
In the discussion surrounding the battery value chain, the spotlight frequently falls upon the sources and provisions of battery materials and components. Nevertheless, an emerging challenge within the automotive sector is notable: the ability to assess batteries to guarantee exceptional performance, durability, and safety.
In a landscape marked by surging customer demands and competitive pressures to enhance range and charging efficiency, engineering teams are grappling with a host of challenges as they navigate the path to product launches. Paramount among these challenges are concerns regarding battery longevity, protracted charging periods, and limited operational range. These aspects present not only hurdles but also opportunities where machine learning and AI can make a significant impact.
Battery testing is extremely complex, involving thousands of design scenarios that all require a very long time to test. As the physics of complex products like batteries become more and more intricate and time-consuming to understand, engineers find themselves in a dilemma; they either conduct excessive tests to cover all possible operating conditions, or run insufficient tests that risk the omission of critical performance parameters.
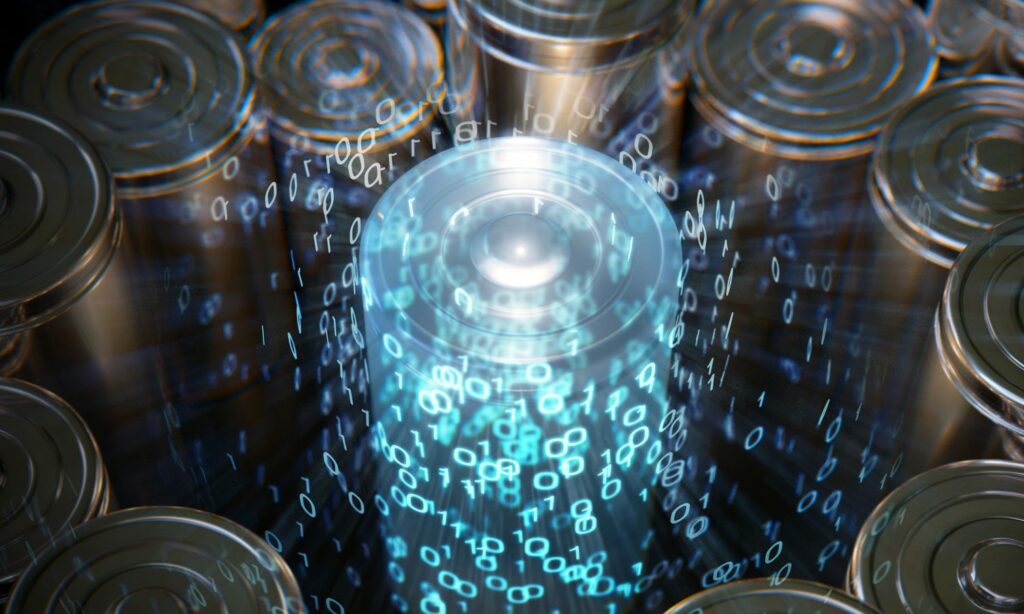
One is time-intensive and risks losing the competitive edge while the other risks brand reputation and customer safety. Through conversations with battery R&D leaders at many global OEMs, it has become evident that engineers are feeling increased pressure when it comes to guaranteeing the thermal performance—as well as the life and health—of EVs in real-world scenarios. Designing these products is a delicate balancing act between multiple parameters, which takes both time and highly advanced tools to achieve.
Every OEM has the challenge of specifying and developing the best battery system for their technology, and they need to go to market with total confidence in how it performs. Engineering a battery pack requires a huge amount of testing—years in some cases—because it’s a complex new technology and in many areas unproven in the field. Balancing key characteristics of an EV battery system such as range, recharge rate, and life-time requires months of validation testing across thousands of scenarios and conditions.
According to the head of testing at a European carmaker, competitive pressures are driving the company to invest more and more time, money, and effort into battery testing. However, a US$30m investment is nothing when you consider the vulnerability to the China-based supply chain. In their words “We have to find independence at any cost.”
Escalating test costs
Many players perform battery tests across thousands of channels, generating terabytes of data per week. The capital costs of building a battery lab capable of testing hundreds of cells are huge, including rows and rows of environmental chambers, battery cyclers, and high-voltage power sources. They’re running out of test stands and don’t know what optimal tests to run, and certainly don’t have the ability to learn from this vast amount of data as quickly as they need. For perspective, the annual cost of testing 300 cells can range anywhere from US$1m to tens of millions depending on the scope and complexity of test scenarios.

The competitive pressures of the market, however, are driving these huge investments to continue at a rapid pace. As more EV models are introduced, OEMs are racing for improvements in battery performance to capture market share as the landscape changes. In addition, European and North American-based manufacturers feel locked into a battery supply-chain dominated by China, which is driving even more urgency and investment.
For battery testing, the fundamental assumption that “more is better” is a fallacy. Expanding test capabilities to test more cells in parallel appears to be a good strategy on the surface, but it doesn’t actually shorten the testing process for ageing, nor does it give a better understanding of cell performance and overall ageing characteristics. A better approach is to focus energy and innovation into a more efficient test plan that explores the right combination of conditions to provide an understanding of battery performance with the fewest test steps and test stands possible.
This is where AI and machine learning come in. Through the ability to learn from data, test engineers can understand behaviour characteristics that are so complex, that without the right tools it is incredibly difficult to decipher. AI that learns from real-world test data is a reliable and effective means for solving the intractable physics of batteries that current simulation and test planning tools don’t efficiently solve.
Applying theory to the commercial world
Earlier this year, researchers at Stanford, MIT, and the Toyota Research Institute conducted experiments applying machine learning techniques to battery testing. The goal was to use AI techniques to reduce the number and duration of tests required to identify the lifecycle of electric vehicle batteries. Traditionally, EV batteries are exhaustively tested to understand the state of the battery health and charge after thousands of charging and discharging cycles under various conditions. Because of the large parameter spaces and high sampling variability, an extremely large number of tests are required to find the expected battery lifetime from a given charge protocol.
By combining multiple AI algorithms, the researchers were able to find the expected lifetime of batteries using a fraction of the tests that traditional methods would require. Where conventional approaches took upwards of 500 days to complete the testing, the teams at Stanford, MIT and Toyota Research were able to apply an iterative, active-learning approach to complete the same result in only 16 days, showing a reduction of nearly 98%.
The Monolith team, looking to validate the Stanford-led research with a commercially available tool, downloaded the data to put the research into practice. Using internal software, Monolith engineers showed reductions in the number of tests required for identifying battery lifetime and finding the optimal charging cycle by 59% and 73% respectively. It should be noted that the approach used general-purpose test optimisation tools designed for analysing and making recommendations on any design space, as opposed to applying a model built specifically for the research data.
Balancing key characteristics of an EV battery system such as range, recharge rate, and life-time requires months of validation testing across thousands of scenarios and conditions
Although data-driven AI models can significantly reduce testing effort and complexity, there are limitations. Self-learning models trained using test data from a given cell chemistry can give a more accurate and complete understanding of performance over different conditions. However, the models are derived from that specific cell chemistry—if the chemistry is changed, new models must be trained.
Relieve stress and power the EV revolution
With these kinds of results, it’s clear that AI is emerging as an intriguing accelerant that can cut time to market significantly for breakthrough products and technologies. Arguably, the biggest opportunity is in validation testing of highly complex, dynamic systems with non-linear behaviour, i.e. EV batteries. By embracing AI and machine learning principles, engineering teams can navigate the intricate challenges of understanding—and validating—the intractable physics of EV batteries more efficiently, leading to streamlined development, optimised designs, and faster time to market.
About the author: Richard Ahlfeld is Chief Executive and founder of Monolith