Over several years, and due to a number of factors, vehicles have become increasingly expensive to run. NimbleFins reported this summer that on average, UK car owners spend over £3,000 (US$3,660) to run their cars each year. Fuel, parts, and repairs have all gone up in price, and many drivers are faced with additional charges for parking, tolls, and for driving in low emissions zones.
On top of this, traffic has become increasingly bad in highly populated urban areas, and this has knock-on effects for the air quality and liveability of cities. Public transport might seem like an obvious answer to these problems, yet the industry has failed to live up to expectations as cars remain the preferred means of transportation for those who can afford one.
Ultimately, this comes down to a matter of reliability. In many respects, the questions that transport providers ask themselves are not so different to those of a car owner: how to extend the life of engines and tyres, how to avoid breakdowns, and how to make journeys more sustainable. In other words, how can they provide a service that passengers can trust at the ‘lowest cost per mile’. The key to ensuring that a service of excellence and the generation of dividends aren’t mutually exclusive is data. With granular data collection and artificial intelligence analysis, operators can finally cut costs while improving the quality of their network.
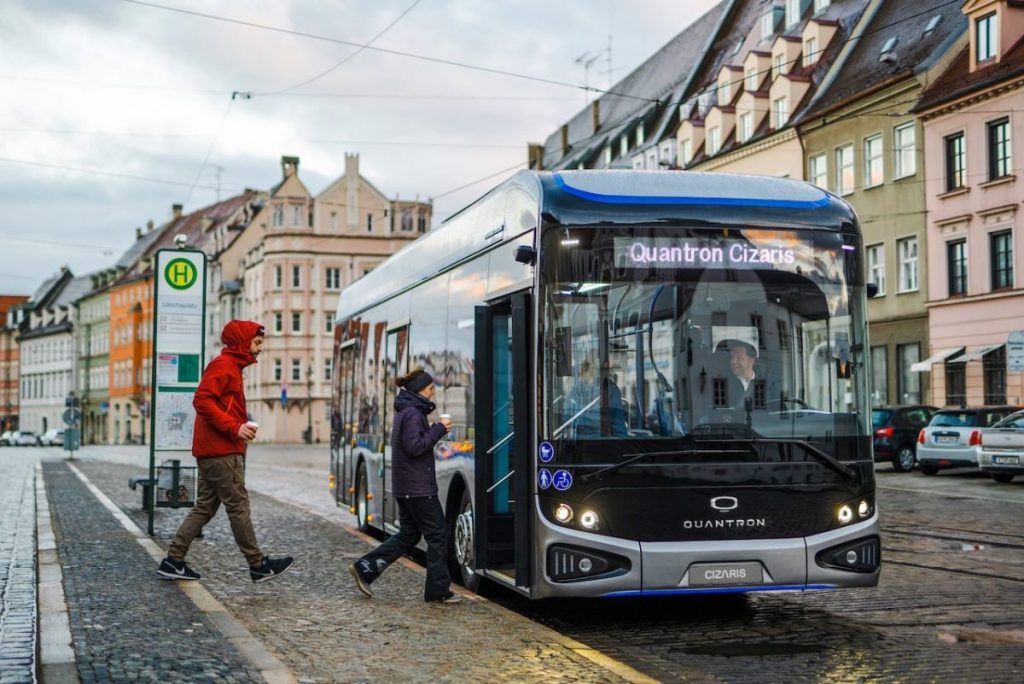
Leveraging data
Over the last decade, data has become the world’s hottest commodity, and decision makers across every industry are asking questions about how to get the most out of the data they collect. Bus operators are no exception: data offers a clear route to improving vehicle reliability and working towards the vision of zero downtime.
The issue of downtime caused by vehicle breakdowns generally stems from a lack of tools to detect and deal with the issue proactively. AI platforms now collect a huge range of readily available data points on vehicle conditions and turn them into real-time actionable insights. This type of early warning system allows transport companies to predict and proactively deal with potential vehicle breakdowns and, as a result, eliminate the issue of unexpected downtime.
The results of predictive maintenance are tangible. Arriva Czech Republic, for example, over the course of a year recorded a 13.5% increase in time between failures, a 66% towing reduction due to vehicle breakdown and total net cost savings of 2% per km.
Despite legacy systems, data silos and internal resistance to change, finding ways to leverage the vehicle data helps bus operators optimise maintenance and operational strategies, and deliver a cost-efficient, reliable service. AI predictive insights can be used to monitor everything from fuel levels to the more complex state of health of major vehicle components and systems, enabling operators to act early and address specific issues before the vehicles hit the road. Data is key in both analysing the existing state of play and predicting outcomes, offering huge advantages to operators.
Route planning
Many bus routes become sub-optimal as cities develop and the needs of inhabitants change. Achieving lowest-cost-per-mile is not only about optimising current services, but about utilising technologies like AI to support route planning by predicting and providing visual data on how new routes would run in the future.
When introducing new bus routes, companies usually rely on people to analyse limited datasets and gauge how popular a given route might be, what the potential high-traffic areas are, where accidents are most likely to occur, what the problems with refuelling might be at certain points along the way. With AI, this can be automated, making roll-out quicker whilst reducing the number of teething problems experienced once buses hit the road. It also frees up time for fleet managers to spend on bigger tasks, such as initiating the rollout of new electric vehicle (EV) fleets.
The key to ensuring that a service of excellence and the generation of dividends aren’t mutually exclusive is data
AI-powered planning and scheduling improves the quality of transit services and can be integrated with predictive maintenance to create a data-driven ecosystem that covers vehicle safety, servicing, and operations. Doing all this in the planning stage saves money down the line, when fewer adjustments need to be made to routes that are already up and running. The result is new services that from the get-go perform at the lowest possible cost to operators, leaving them money to re-invest in improving services even more.
Greener futures
Public transport has always been the greener option when it comes to travelling, but it has not been successful in swaying the number of people still choosing to own a car. In fact, the total number of licensed vehicles has increased in all but two years since the end of the Second World War.
Although governments around the world have introduced reforms over the years to reduce the impact of high car ownership on carbon emissions, not enough has been done to encourage alternative modes of transport. People have simply felt there is no alternative option, despite many regions boasting expansive bus and rail networks. The unreliable nature of public transport has led to a generation of travellers lacking trust in operators.
However, as people strive to adopt to a more sustainable lifestyle, and as the costs of car ownership rise, bus operators now have the opportunity to rebuild that trust with consumers. In order to make the world a greener place with cleaner air and more sustainable vehicles, it’s imperative that public transport becomes more attractive to the average person.
There is no mutual exclusivity between bus operators striving to become more internally efficient and delivering a better service to customers. In fact, the latter simply cannot be accomplished without an ongoing commitment from operators to invest in new technologies that free up staff and create new pools of cash that can be reinvested. Going green is non-negotiable for bus operators, with governments already making their intentions clear, so there has never been a better time for delivering reliable services backed by AI-powered analytics. By investing in best-in-class batteries and the right AI platforms, bus operators are cementing the place of public transport in the heart of the smart cities of the future.
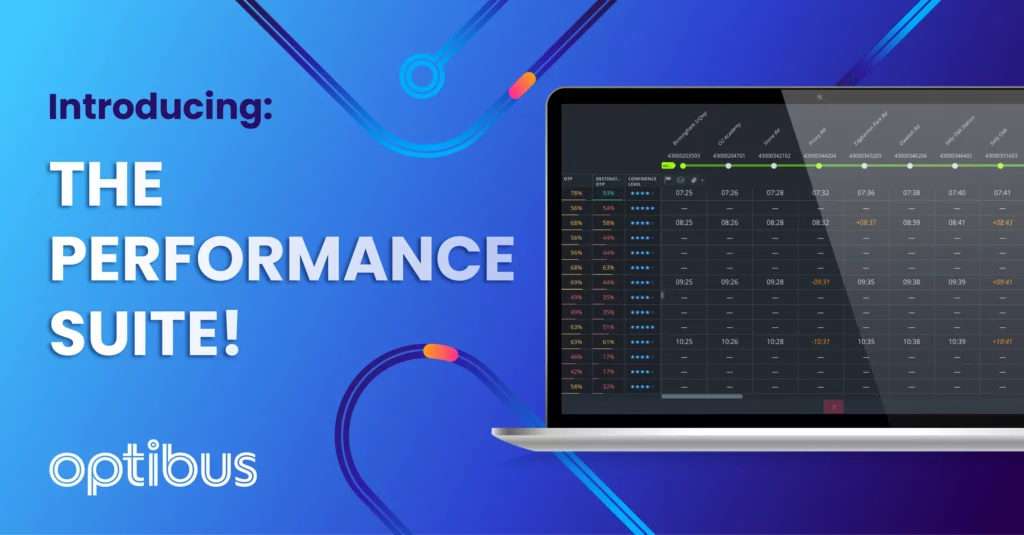
Fostering partnerships
In the UK, up to 25% of maintenance issues across all bus operating systems could be prevented or handled in a more cost-effective way if operators had the technology to predict and mitigate those issues early on. For specific vehicle systems, the estimated percentage of avoidable failures may be as high as 70%.
Decision makers in public transport are now starting to see that in order to be successful, they need to accelerate the integration of historical data, artificial intelligence, and vehicle health monitoring to avoid unforeseen maintenance issues arriving in the middle of service. Operators are the transport experts, and data scientists are the data experts— so collaboration is more important than ever.
Optibus, a company with a leading software platform for planning, operating, and optimising public transportation, was the first company of its kind to start utilising predictive maintenance technology to solve major operational and vehicle maintenance challenges in public transport with artificial intelligence. This is indicative of the new era in public transport. As operators look to explore new data-first avenues, the key question for them is around finding partners that can help them rebuild trust with customers, who want reliable and efficient public transport.
About the author: Giorgio Sarno is Data Scientist Lead at Stratio